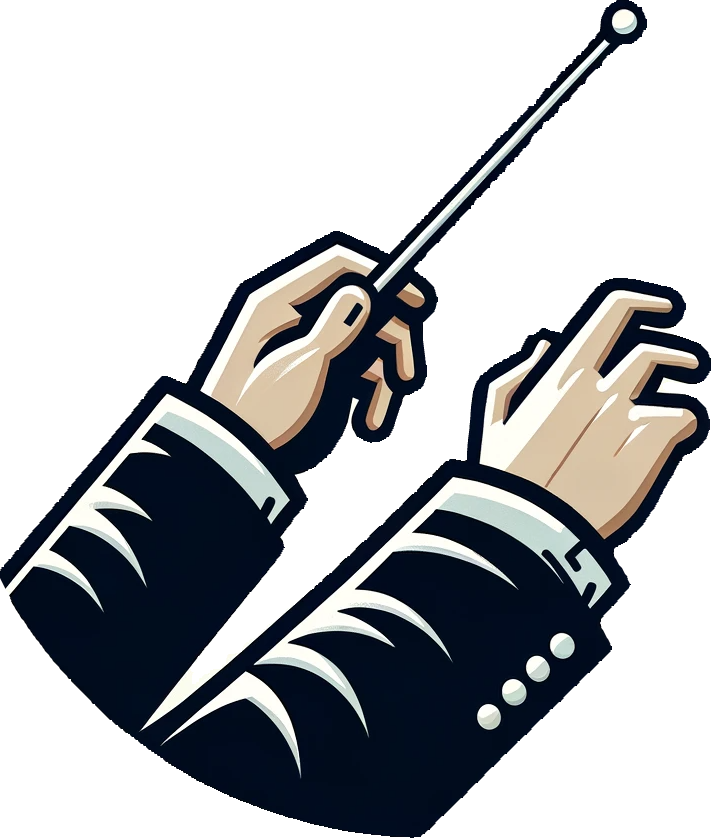
BATON: Aligning Text-to-Audio Model with Human
Preference Feedback
1 Tsinghua University, 2 Huawei Technologies Co., Ltd
Abstract
With the development of AI-Generated Content (AIGC), text-to-audio models are gaining widespread attention. However, it is challenging for these models to generate audio aligned with human preference due to the inherent information density of natural language and limited model understanding ability. To alleviate this issue, we formulate the BATON, a framework designed to enhance the alignment between generated audio and text prompt using human preference feedback. Our BATON comprises three key stages: Firstly, we curated a dataset containing both prompts and the corresponding generated audio, which was then annotated based on human feedback. Secondly, we introduced a reward model using the constructed dataset, which can mimic human preference by assigning rewards to input text-audio pairs. Finally, we employed the reward model to fine-tune an off-the-shelf text-to-audio model. The experiment results demonstrate that our BATON can significantly improve the generation quality of the original text-to-audio models, concerning audio integrity, temporal relationship, and alignment with human preference.
Pipeline
Figure 1: BATON integrates three modules: An audio generation unit using LLM-augmented prompts, with human-scored annotations (left); A reward model trained on synthetic data to emulate human alignment preference (center); A fine-tuning mechanism that enhance the original generative model using reward model combined human-labeled and pre-training datasets (right).
Generation of Audio Captions Using LLM
Examples of Integrity Captions:
Labels Given to ChatGPT | Captions Generated by ChatGPT |
---|---|
"Baby cry, infant cry", "Waves, surf" | A baby cries while waves crash onto the shore. |
"Canidae, dogs, wolves", "Rustle" | Dogs and wolves howl and bark, rustling leaves in the background. |
Examples of Temporal Captions:
Labels Given to ChatGPT | Captions Generated by ChatGPT |
---|---|
"Crying, sobbing", "Toilet flush", "Female singing" | Young child crying at first, then a toilet flushes, as a woman's singing begins. |
"Rain on surface", "Helicopter", "Engine starting" | Rain on a surface, followed by a helicopter, and then an engine starts. |
Comparison of Generated Results
Integrity Task:
BATON(ours) | TANGO(Full-FT-Audiocaps) | AudioLDM2(Full-Large) |
---|---|---|
![]() |
![]() |
![]() |
Footsteps and a croaking frog |
||
![]() |
![]() |
![]() |
A loud bang followed by an engine idling loudly |
Temporal Relationships Task:
BATON(ours) | TANGO(Full-FT-Audiocaps) | AudioLDM2(Full-Large) |
---|---|---|
![]() |
![]() |
![]() |
Speech and then a sneeze and laughter |
||
![]() |
![]() |
![]() |
A man talking as music is playing followed by a frog croaking |
More Results of BATON
Integrity Task:
Caption | BATON (sample = 2) | |
---|---|---|
A dog whimpering constantly then ultimately growls |
||
A motor hums softly followed by spraying |
||
Running then spraying |
Temporal Relationship Task:
Caption | BATON (sample = 2) | |
---|---|---|
A man talking then laughing as ducks quack |
||
A man speaking as footsteps walk on grass while insects are buzzing |
||
A man briefly speaks followed by a sawing sound, and then starts talking again |
BibTeX
@article{liao2024baton, title={BATON: Aligning Text-to-Audio Model with Human Preference Feedback}, author={Liao, Huan and Han, Haonan and Yang, Kai and Du, Tianjiao and Yang, Rui and Xu, Zunnan and Xu, Qinmei and Liu, Jingquan and Lu, Jiasheng and Li, Xiu}, journal={arXiv preprint arXiv:2402.00744}, year={2024} }
Acknowledgement
Thanks for the excellent open-source project demo page template provided by AudioLDM.